Larry Summers — AGI and the Next Industrial Revolution (#159)
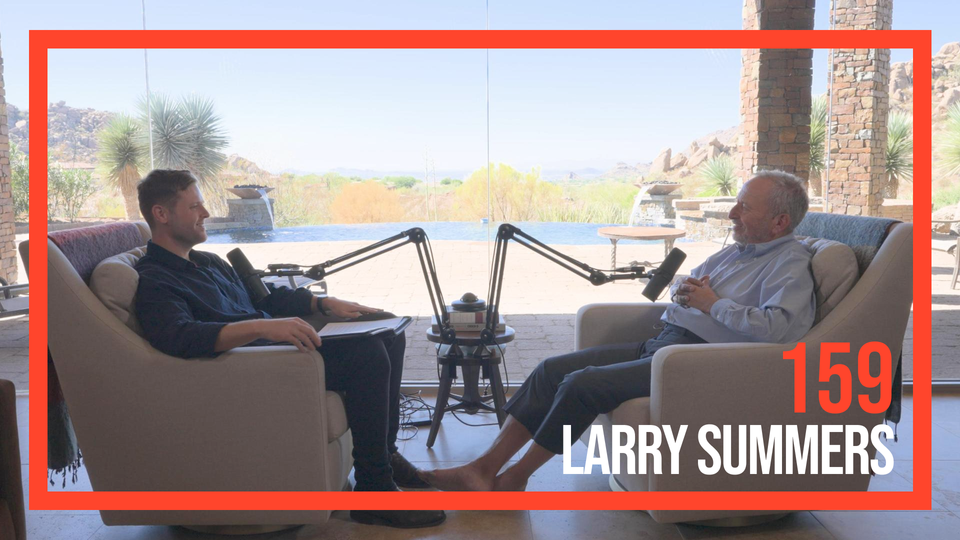
Larry Summers is a former US Treasury Secretary (1999-2001), Chief Economist at the World Bank (1991-1993), and Director of the National Economic Council under President Obama (2009-2010). He also served as President of Harvard University (2001-2006).
Currently, he is the Charles W. Eliot University Professor at Harvard University, and he sits on the board of directors at OpenAI, one of the fastest-growing companies in history.
Video
Transcript
JOSEPH WALKER: Today, it's my great honour to be speaking with Larry Summers. Larry is arguably the preeminent American economic policymaker of his generation. He was a Secretary of the Treasury, among many other roles, and he's currently on the board at OpenAI, among many other roles. Larry, welcome to the podcast.
LARRY SUMMERS: Good to be with you.
WALKER: In this conversation, I want to focus a lot on the economic implications of AI.
If, as many serious people think, AI is likely to induce a step-function change in human economic growth, getting to chat with you in 2024 feels a little bit like an interviewer getting to speak with Adam Smith in the early decades of the Industrial Revolution—except I feel like I'm in a much more privileged position because I think you know a lot more about what's happening in San Francisco than Smith knew about what was going on in Manchester and Birmingham.
So, first question: you joined the board at OpenAI about a year ago, and that means if OpenAI succeeds in creating artificial general intelligence in the next few years, as it's attempting to do, you'll be one of nine people in the room who determines whether that has happened.
I know you've been thinking at least about the economic implications of the technology for several years, but perhaps you hadn't thought so much about the technology itself, about deep learning, until you joined the board.
So I'm just generally interested, how does someone like Larry Summers go about getting up to speed on a new topic? With respect to the technology itself, what kind of things have you been reading? What kinds of people have you been speaking to? What kinds of learning strategies have you been employing?
SUMMERS: Look, I think this is a fundamentally important thing. I think that the more I study history, the more I am struck that the major inflection points in history have to do with technology. I did a calculation not long ago, and I calculated that while only 7% of the people who've ever lived are alive right now, two-thirds of the GDP that's ever been produced by human beings was produced during my lifetime. And on reasonable projections, there could be three times as much produced in the next 50 years as there has been through all of human history to this point. So technology, what it means for human productivity—that's the largest part of what drives history. So I've been learning about other technological revolutions.
I had never been caused to think appreciably about the transition thousands of years ago from hunter-gatherer society to agricultural society. I've thought about the implications of the Renaissance, the implications of the great turn away from a Malthusian dynamic that was represented by the Industrial Revolution. So the first part of it is thinking about technology and what it means in broad ways.
The second is understanding, not at the level of a research contributor to the science, but at the level of a layperson, what it is that these models are doing—what it means to think about a model with hundreds of billions of parameters, which is an entirely different, new world for somebody who used to think that if he estimated a regression equation with 60 coefficients, that was a really large model.
So I've been watching blogs, listening to YouTube tutorials, spending my time talking with people at OpenAI to try to get an understanding of the technology and what's involved in the science of the technology.
At one stage, when I expressed this interest and Sam Altman asked me, "Do you want to learn to program these things?" I said, "No, I'm too old for that.”
I want to get to the kind of understanding that you can get to of physics if you're not willing to learn the mathematics of tensors, the kind of understanding that you can get to here, short of being a person who can actually execute.
And then I've tried to read literature and talk to people who are engaged in application and are prepared to speculate about what kind of applications are likely to be possible at some point in the future.
So it's a combination of understanding relevant historical moments, understanding the stuff of the technology, and thinking about people who are engaged in the relevant kind of application.
I suppose it's a little bit like if you were present at the moment when nuclear became possible, you'd want to understand previous moments of staggering new destructive technology. You'd want to talk a lot with the physicists who were involved, and you'd want to talk to military strategists, doctors who had potential uses for radiation, those involved in the energy industries who might want to think about the implications of inexpensive energy not coming from fossil fuels.
Of course, I think that this technology potentially has implications greater than any past technology, because fire doesn't make more fire, electricity doesn't make more electricity. But AI has the capacity to be self-improving.
WALKER: On the technology itself, so maybe you're not going to learn how to code up a transformer or whatever, but do you recall some of the specific videos you've watched or things you've read that were especially helpful?
SUMMERS: You know, I think that I don't want to get involved in… since I don't remember precisely which of them were more proprietary and which of them were not. But I think there are a number that have come out of OpenAI, but they've come out of other places as well. Tutorials that have been written on what these parameters are.
Susan Athey and Sendhil Mullainathan, among economists, have written powerfully about these models in ways that are accessible to people like me, whose initial and early trainings were in econometrics and statistical inference. And so I would mention their writings as things that are particularly relevant.
WALKER: And just quickly, since you joined the board at OpenAI, roughly how many hours per week have you been spending on OpenAI-related stuff?
SUMMERS: I think it varies, but a day a week would be in the range.
And some of that has been trying to come up to speed with understanding the technology. Some of that has had to do with a company that has mushroomed in scale and that has developed large revenue streams and market value probably faster than any company in history, has all sorts of governance challenges and issues, and that has been part of my concern and remit as well.
WALKER: If you think of all the various bottlenecks to scaling AI—data, chip production, capital, energy, et cetera—which one strikes you as the most underrated at the moment?
SUMMERS: Well, I would not underestimate the fact that there are substantial questions around imagination and things still happen that surprise people. And so ultimately, I suspect that when the history of this is written after it's been successful, new great insights about ways to strengthen reasoning capacity, ways to use compute more efficiently, ways to generate information that can be a basis for training... I would emphasise ideas and having more of them more quickly that can come to application is, I think, something that's very important.
In the terms which you asked the question, I suspect that for the near term, the constraint is likely to be on compute and on access to chips that can be used both in training and inference in these models.
I think if you take a somewhat longer run view, I suspect that energy is likely to be the larger constraint. But probably sophisticated chips is the nearer term limiting factor on which I'd focus.
WALKER: I want to elicit one more premise before we move on to talking about the economic implications. Approximately what share of time do today's AI researchers spend on tasks that AI will be doing for them in five years? (Based on your conversations with technologists.)
SUMMERS: I don't know, but if the answer were less than 25%, I'd be quite surprised. And if the answer were more than 75%, I'd be quite surprised. But it's very hard for me to estimate in between.
And in a way, it depends on how you exactly define the tasks. You know, ordering lunch is part of our day, managing our lives is part of our day, managing routine corporate interactions, scheduling, is part of our day, and that stuff will obviously be among the first stuff where there will be substitution.
But even in tasks that are closely defined as research, I think the capacity of AI to program and to create software is likely to be a very substantial augmenter of what software engineers do.
WALKER: So the range of opinions is 25% to 75% of AI research?
SUMMERS: I'm not sure whether that's the range of opinions. The range of opinions as to the best guess might be smaller than that. But I think the range of uncertainty about what the reality is probably very wide at this point, but with a pretty high floor.
WALKER: Got it. So maybe about 50% of AI research itself might be automated in five years?
SUMMERS: I don't want to... I want to preserve the sense of very great uncertainty.
WALKER: Fair enough. So let's talk about the economic implications of AI. First, a somewhat tangential question. If we take the last 150 years of real US GDP per capita growth, it's grown at about 2% per year. It's been remarkably steady. And the biggest interruption to that was obviously the Great Depression, where GDP plunged about 20% in four years. But then it just quickly resumes its march of about 2% per year. What do you think is the best explanation for the remarkable steadiness of US growth?
SUMMERS: Well, I think it's been a little more complicated than that because I think you have to start by thinking about growth as the sum of workforce growth and productivity growth. And there's been fluctuation in both of those things.
When I first started, begun started studying economics as a kid in the 1960s, people thought that the potential GDP growth of the United States was approaching 4% because they thought at that time that population and labour force growth would run at about 2%, and they thought that productivity growth would run at 2%.
Today, we have rather more modest conceptions because labour force growth is likely to be much slower, given that women on average are now having less than two children, that immigration is somewhat limited, and that the very large wave of increased labour force growth that came about as it became presumptive for young and middle-aged women to be in the labour force, that was a one-time event. So labour force growth: slower than it used to be.
Productivity growth was much faster from 1945 to 1973 than it was subsequently. There was a very good decade from the early mid-90s to the early mid-decade of the aughts. But other than that, productivity growth has been running distinctly south of 1%, at least as we measure it.
So I'm not sure there's any God-given law that has explained why it has been relatively stable, because the things underneath it have been fluctuating a fair amount. But I suspect that if one was looking to theorise about it would be that for societies at the cutting edge, like the United States, there's only so much room for the creation and application of new technology, and that labour force growth and capital accumulation associated with labour force growth have an inherent stability to them.
WALKER: Okay, so to make sure I understand, for frontier economies, it's much more likely that there's a kind of endogenous story that's explaining why growth's been so steady, relating to population growth maybe counterbalancing ideas getting harder to find, or something like that?
SUMMERS: Yeah, I don't want to overdo… I think your statement, respectfully, Joe, probably overstated just how much stability there has been from period to period and from decade to decade.
And of course, if you look at non-frontier economies, they often, or not usually, but in a number of highly prominent cases—the Asian countries, most of which are concentrated in Asia—have had periods of extremely rapid growth that came in part from integrating into the global economy and developing technological capacity as they did that.
WALKER: Right. So if we take the long view and look at gross world product over many thousands of years, growth rates have been increasing over time. How likely is it that AI initiates a new growth regime with average growth that’s, say, ten times faster than today?
SUMMERS: I think the kind of growth that followed the Industrial Revolution was probably unimaginable to people before the industrial revolution. And I think even the kind of growth that followed the Renaissance, that can perhaps be dated to the 1500s, probably seemed implausible to people beforehand.
So I hesitate to make definitive statements. My instinct is that substantial acceleration is possible. I find 10x (to be growth at a level where productivity doubles every four years) to be hard to imagine.
There are certain things that seem to me to have some limits on how much they can be accelerated. It takes so long to build a building, it takes so long to make a plan. But the idea of a qualitative acceleration in the rate of progress has to be regarded, it seems to me, as something that's very possible.
WALKER: Some people think that AI might not only deliver a regime of much faster economic growth, but might actually instigate an economic singularity where growth rates are increasing every year. And the mechanism there would be that we automate A in our production function, and so we have this feedback loop between output and R&D being increasingly automated. What do you think is the best economic argument for believing that ever-increasing growth rates won't happen with AI? Is it some kind of Baumol's cost disease argument, where there are still going to be some bottlenecks in R&D that prevent us from getting those ever-increasing growth rates?
SUMMERS: I would put it slightly differently. I think I would put it that, in a sense, sectors where there's activities where—and this is in a way related to your Baumol comment—there is sufficiently rapid growth almost always see very rapidly falling prices. And unless there's highly elastic demand for them, that means they become a smaller and smaller share of the total economy. So we saw super rapid growth in agriculture, but because people only wanted so much food, the consequence of that was that it became a declining share of the economy. And so even if it had fast or accelerating growth that had less and less of an impact on total GDP growth. In some ways we're seeing the same thing happen in the manufacturing sector where the share of GDP that is manufacturing is declining.
But that's not a consequence of manufacturing's failure. It's a consequence of manufacturing's success.
A classic example was provided by the Yale economist Bill Nordhaus with respect to illumination. The illumination sector has made vast progress, 8, 10 per cent a year for many decades. But the consequence of that has been that on the one hand, there's night little league games played all the time in a way that was not the case when I was a kid. On the other hand, candlemaking was a significant sector of the economy in the 19th century, and nobody thinks of the illumination sector as being an important sector of the economy [today].
So I think it's almost inevitable that whatever the residuum of activities that inherently involve the passage of time and inherently involve human interaction, it will always be the case that 20 minutes of intimacy between two individuals takes 20 minutes.
And so that type of activity will inevitably become a larger and larger share by value of the economy. And then when the productivity growth of the overall economy is a weighted average of the growth individual sectors, the sectors where there's the most rapid growth will come over time to get less and less weight.
WALKER: Right. So I want to talk about how AI might be applied to enable economic policymakers. And I want to speak first about developing countries. So, assume that we do get AGI. I wonder how much that might be able to help economic policymakers in developing countries. Maybe you could interpret the success of the Asian Tiger economies—where they were getting consistent 7.5% GDP growth per year—as existence-proof that much better economic policymaking can translate into massive increases in GDP. But on the other hand, there are these constraints, like social and political constraints, which might be more important. So how much do you think AI would be able to enable greater economic growth in developing countries through helping policymakers make better decisions?
SUMMERS: Well, I think the ability to import knowledge and apply that knowledge and expertise pervasively is something that is very important apart from economic policy. It was really hard for the United States to learn a lot of what was known in Britain about how to make a successful textile factory in the early 19th century. With AI, what's known anywhere is likely to be known everywhere to a much greater extent than is true today—that more-rapid transmission of knowledge is, I think, likely to be the most important positive in terms of accelerating development.
Certainly, there are hugely consequential and difficult choices that developing country policymakers make, whether it's managing monetary policy or, probably even more consequentially, strategic sectoral policies about which sectors to promote.
To the extent that AI will permit a more accurate and full distillation of past human experience and extrapolation to a new case, I think it's likely to contribute to wiser economic policy, which permits more rapid growth.
WALKER: Moving to the US, take the Fed, for example. How much better could monetary policy be if the Fed had AGI? Could we massively reduce the incidence of financial and macroeconomic instability? Or are those things subject to chaotic tipping points that just aren't really amenable to intelligence?
SUMMERS: I think it's a very important question. The weather and the equations that govern weather are susceptible to chaotic dynamics, and that places sort of inherent limits on weather forecasting. Nonetheless, we're able each decade to go one day longer and have the same quality forecast that we had in the previous decade. So the five-day forecast in this decade is like the four-day forecast was a decade ago, or the three-day forecast was two decades ago.
So I suspect we are far short of some inherent limit with respect to economic forecasting.
I'm not certain, because there's a fundamental difference between economic forecasting and weather forecasting, which is the weather forecast doesn't affect the weather, but the economic forecast does affect the economy.
But my guess is that we will be able to forecast with more accuracy, which means we will be able to stabilise with more accuracy, and that should lead to better policies. And it may be that we will find that, to take a different sort of natural world problem, AI will improve the field of seismology, earthquake prediction, which involves predicting rare convulsive events. And it may be that it will aid in predicting financial crashes and evaluating bubbles. And all of that would obviously also contribute to stabilisation policy. So I would expect meaningful progress to come over time.
I would caution as a very general rule, Joe, that things take longer to happen than you think they will, and then they happen faster than you thought they could. And so I would hesitate to assume that these benefits are going to be available to us immediately, just as I would hesitate to think that we're not going to make progress from where we are now.
WALKER: There'll probably be a J-curve for AI.
So, retrospectively, how much would having AGI have helped economic policymakers in the Obama administration during the financial crisis and Great Recession? Because if I think about that time, what was scarce wasn't so much intelligence, but what I would describe as constraints of human social organisation. Two examples. Firstly, cram-down legislation wasn't passed, not because people didn't know it would be helpful, but because the Obama administration couldn't muster the requisite 60 votes in the Senate. Or another example: policies to convert debt to equity weren't implemented, not because economists didn't realise that wouldn't have helped, but because the administration lacked the sort of state capacity to negotiate and track those contracts over time. So how much would AGI have helped you during the financial crisis and Great Recession? Or were the constraints things that, again, weren't really amenable to intelligence?
SUMMERS: I'm not sure that in either of these cases, it's quite as simple as you suggest. Depending on how cram-down legislation was structured, it could have set off a wave of bankruptcy-type events that would have had moral hazard consequences and exacerbated the seriousness of the financial crisis. And so that kind of uncertainty was one of the things that held back and slowed the movement of that legislation, and similarly with respect to various other schemes.
But in general, it is easier to reach solutions where the epistemology is clear. And I would think that better knowledge of all the aspects of the financial crisis and better and more shared understandings of the causal mechanisms, which I think comes from tools that promote better research, would likely have been to lead to better solutions.
On the other hand, I think in retrospect, most people feel that the fiscal stimulus provided by the Obama administration was too small. In my judgement (and I think the [judgement of] people who were closest to the event), that did not reflect a misguided analytical judgement by the Obama administration; it reflected the political constraints of working to get rapid progress through Congress.
Now, if there had been better economic science, and so it had been clear what the right size of stimulus was, and the argument was less arbitrary, people would have been more likely to have been prepared to politically support the right thing.
So I think there is a contribution. I like to say that it's no accident, Joe, that there are quack cures for the common cold and some forms of cancer, but no quack cures for broken arms or strep throat.
And that's because when there's clear and definitive knowledge and understanding, then people rally around behind that. But when there isn't an expert scientific solution that works, that's when you get more debate, more volatility of approach, perhaps more flaky solutions. And I think better artificial intelligence, over time is likely to drive greater understanding, and that will contribute to better outcomes.
WALKER: Interesting. Some final questions on the geopolitical implications of AI and governance. We don't have to spend too much time on this, but you drew the analogy earlier to the technology of nuclear energy and atomic weapons. I had an interview with Richard Rhodes last year, and he mentioned that the Manhattan Project was infiltrated by Russian spies almost immediately. Stalin had about 20 to 30 people in the Manhattan Project over the course of the war. Klaus Fuchs was literally giving the blueprints for the implosion device to Stalin, indirectly, and he was one of the scientists on the project. There's no way the CCP isn't already infiltrating major AI labs in the US and UK and stealing their IP, right?
SUMMERS: Look, I think that this is going to be an important area for us, for everybody to think about going forward. And thinking about the security and thinking about the importance of American leadership is, I think, a very large issue.
On the one hand, a certain amount of open flow of information is what drives our progress and is what keeps us ahead.
On the other hand, there is a tension between the preservation of secrecy and the open flow of information.
What's pretty hard to judge is what kinds of things you can learn by spying on and what kinds of things you can't. And, you know, I use the example of the difficulties that the Americans had emulating British textile technology in the 1800s. It's not that they couldn't get blueprints of the British factories.
It's that a blueprint wasn't really enough to figure out how to make a factory work effectively. And there are all sorts of things like that. So what the right way to manage the security aspects is, after all, openness and the sense of our advantage in developing new technologies relative to what the more closed Soviet Union had (that, on most readings of history, contributed to our winning the Cold War in the 1980s).
So I would recognize the overwhelming importance of security issues. But what kinds of leaks we should do. how much to control, I think are very complex questions, and not all proposals that are directed at restricting the flow of information are necessarily desirable because they may so chill our own capacity to make progress.
WALKER: I have many follow-up questions on that, but in the interests of time I'll jump to my next question. Say we wanted to create a “Larry Summers checklist” of criteria or thresholds for when artificial intelligence should be nationalised, should become a government project, what would that checklist contain?
SUMMERS: You know, I'm not certain that I'd quite accept the premise of the question that at some point it should be nationalised. I mean, there have been immense implications of powerful computing. If you think about it, powerful computing over the 60 years since the 1960s has transformed everything. There's nothing military we do that doesn't depend upon computing; an automobile is a very complex computing device with hundreds and thousands of chips. Computing is central to national security. But it never would have been a good idea to nationalise computing.
Should there be some things that are nationalised and should the government have a capacity to produce in certain areas? Yes.
But if you think about our history, if you think about how we put a man on the moon, we didn't nationalise that project, though the government exerted huge degrees of control over how that project was going to take place.
So I am open to the idea that there are certain things that government should nationalise. But I think framing the principal way that governments take responsibility or nurture the development for national security of technology is to nationalise them is, I think, an ahistoric view.
WALKER: Which parts of the production line for AI are the things that would be the biggest candidates for nationalisation?
SUMMERS: I don't feel like I have a good sense of that. Again, I would come back to computing where it doesn't feel like we've nationalised much of anything, but we've managed it, in the fullness of it all, really very very well.
So, I don't want to rule out that there would be things that should be nationalised at all, but I don't want to lean into that as a principal policy response either.
WALKER: Of all the US presidents, you've worked most closely with Bill Clinton, you probably have the best model of him. As we get closer, potentially, to artificial general intelligence, what's your model of… Say, Bill Clinton was president, how would he be thinking about the governance aspects of that problem?
SUMMERS: Well, I worked very closely with both Bill Clinton and Barack Obama, and I think they both were enormously thoughtful, and I think they both recognized that complicated problems required evolutionary rather than revolutionary solutions, that they needed to be approached through multiple channels, and that, in some ways, seeds needed to be planted, and then one needed to see what the best kind of solution was.
But I think government needs to be very familiar with what is going on, have close relationships with the major actors. But I think you need to be very careful that establishing one particular structure to channel things in a particular direction, if that turns out not to have been the right direction, can be very costly.
WALKER: So you want a portfolio approach. Penultimate question: if OpenAI changes its structure from a partnership between a nonprofit and a capped for-profit, to a public benefit corporation, how do its incentives change?
SUMMERS: I think that a public benefit corporation can have all the incentives to responsible stewardship that a not-for-profit can, and that indeed, the history of not-for-profit hospitals and a variety of other not-for-profit structures suggests that they can be very much dominated by the commercial incentives of those who act within them. So I don't think of the possibility of moving to a benefit corporation as reflecting any desire to move away from public interest-type considerations, rather a way to reflect existing not-for-profit law, which limits the ability of not-for-profits to control for-profit entities, and to reflect also the need to have vehicles that can be credible capital raisers to pursue a public interest mission.
WALKER: Final question. So you operate in two relevant worlds. One is the world of technologists, which you have contact with through the board. The other is the world of academic economists, who, on the whole, don't seem overly convinced of AI's extraordinary economic potential. For example, Daron Acemoglu, who won the Nobel Prize a couple of days ago, has this paper where he predicts that AI will deliver productivity increases of only about 0.6% over ten years. How do you explain this discrepancy? And what does the economics profession seem to be missing about AI?
SUMMERS: I have huge respect for Daron, but I don't find his analysis convincing on this. He leaves out entirely in that analysis the possibility that we will have more rapid scientific progress, more rapid social-scientific progress, or better decision-making because of artificial intelligence.
So his analysis seems to me to have the character of the analysis that was done by IBM that concluded that the worldwide market for computers would be five mainframes, or the analysis that was done by AT&T at one stage: they couldn't imagine a demand for as many as a million cell phones globally.
WALKER: Larry, it's been a great honour speaking with you. I know you now have to go to another call, but thank you so much for being so generous with your time.
SUMMERS: Thank you.